Poker Neural Net
Expert-Level Artificial Intelligence in Heads-Up No-Limit Poker
It is shown that, al- though neural networks might be suitable for creating poker agents, a reasonable level of poker will not be achieved with only the most ele- mental features. It is suggested that to achieve a better poker agent, some form of opponent modelling is needed.
- The training data are game histories, and the output is a neural network that estimates the gain/loss of each legal game action in the current game context. We believe that this data-driven approach can be applied to a wide variety of poker games with little game- specific knowledge.
- The prediction 1 is served as one of input features for second neural network. Other features including odd or even, prime property and equality are standardized by the StandardScaler function. Train the second neural network using backpropagation algorithm. The second neural network has 200x100x50 neurons 3 hidden layers.
- Nicolai and Hilderman presented several algorithms for teaching agents how to play no-limit Texas Hold’em Poker using a hybrid method known as evolving neural networks. Evolutionary algorithms mimic natural evolution and reward good decisions while punishing less desirable ones. The system has 35 inputs, 20 hidden nodes and 3 outputs.
- The objective is to train the neural network to predict which poker hand do we have based on cards we give as input attributes. First thing we need for that, is to have a data set.
Links
Twitch YouTube Twitter
Downloads & Videos Media Contact
DeepStack bridges the gap between AI techniques for games of perfect information—like checkers, chess and Go—with ones for imperfect information games–like poker–to reason while it plays using “intuition” honed through deep learning to reassess its strategy with each decision.
With a study completed in December 2016 and published in Science in March 2017, DeepStack became the first AI capable of beating professional poker players at heads-up no-limit Texas hold'em poker.
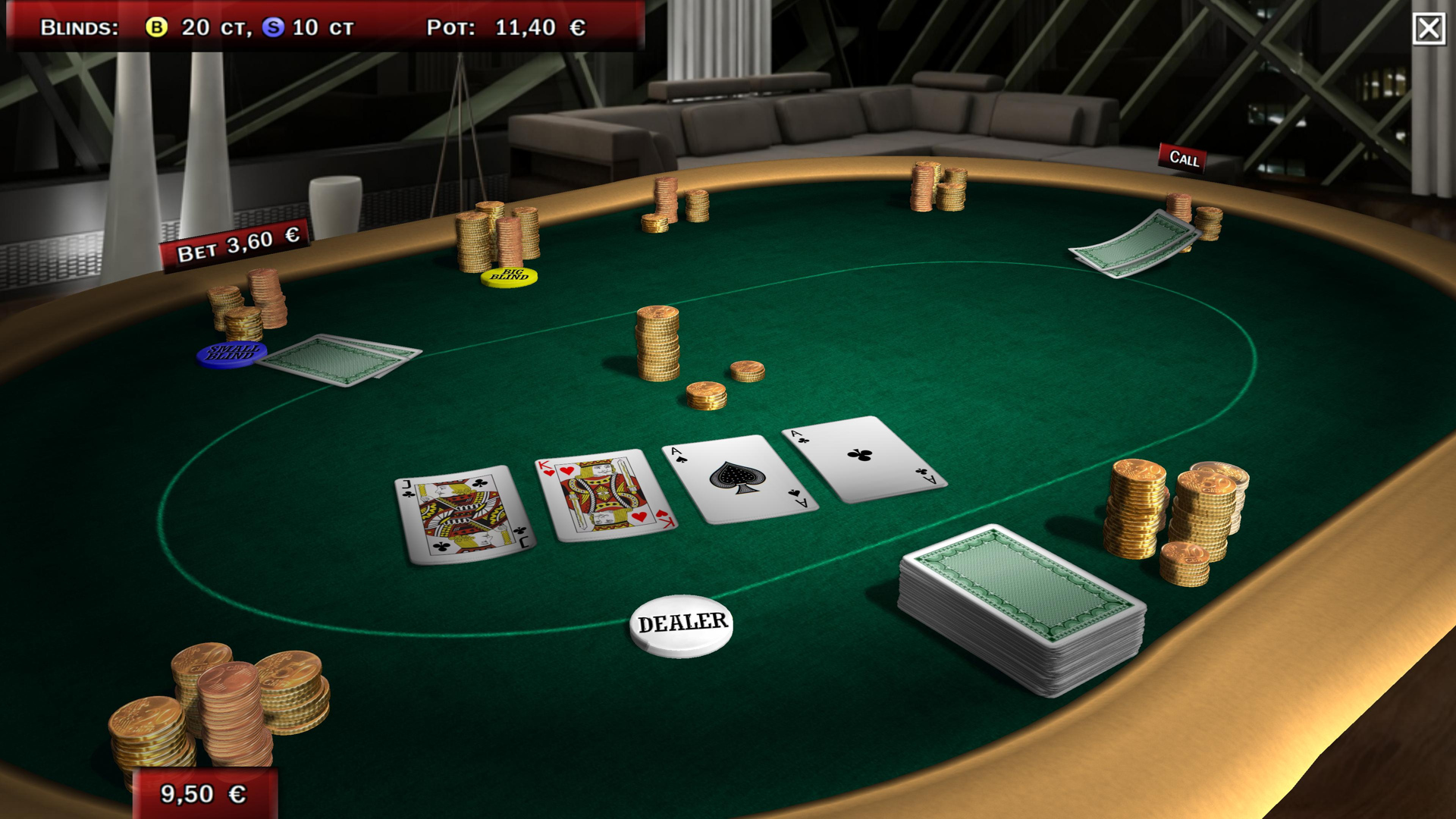
DeepStack computes a strategy based on the current state of the game for only the remainder of the hand, not maintaining one for the full game, which leads to lower overall exploitability.
DeepStack avoids reasoning about the full remaining game by substituting computation beyond a certain depth with a fast-approximate estimate. Automatically trained with deep learning, DeepStack's “intuition” gives a gut feeling of the value of holding any cards in any situation.
DeepStack considers a reduced number of actions, allowing it to play at conventional human speeds. The system re-solves games in under five seconds using a simple gaming laptop with an Nvidia GPU.
The first computer program to outplay human professionals at heads-up no-limit Hold'em poker
In a study completed December 2016 and involving 44,000 hands of poker, DeepStack defeated 11 professional poker players with only one outside the margin of statistical significance. Over all games played, DeepStack won 49 big blinds/100 (always folding would only lose 75 bb/100), over four standard deviations from zero, making it the first computer program to beat professional poker players in heads-up no-limit Texas hold'em poker.
Games are serious business
Don’t let the name fool you, “games” of imperfect information provide a general mathematical model that describes how decision-makers interact. AI research has a long history of using parlour games to study these models, but attention has been focused primarily on perfect information games, like checkers, chess or go. Poker is the quintessential game of imperfect information, where you and your opponent hold information that each other doesn't have (your cards).
Until now, competitive AI approaches in imperfect information games have typically reasoned about the entire game, producing a complete strategy prior to play. However, to make this approach feasible in heads-up no-limit Texas hold’em—a game with vastly more unique situations than there are atoms in the universe—a simplified abstraction of the game is often needed.
A fundamentally different approach
DeepStack is the first theoretically sound application of heuristic search methods—which have been famously successful in games like checkers, chess, and Go—to imperfect information games.
At the heart of DeepStack is continual re-solving, a sound local strategy computation that only considers situations as they arise during play. This lets DeepStack avoid computing a complete strategy in advance, skirting the need for explicit abstraction.
During re-solving, DeepStack doesn’t need to reason about the entire remainder of the game because it substitutes computation beyond a certain depth with a fast approximate estimate, DeepStack’s 'intuition' – a gut feeling of the value of holding any possible private cards in any possible poker situation.
Finally, DeepStack’s intuition, much like human intuition, needs to be trained. We train it with deep learning using examples generated from random poker situations.
DeepStack is theoretically sound, produces strategies substantially more difficult to exploit than abstraction-based techniques and defeats professional poker players at heads-up no-limit poker with statistical significance.
Poker Neural Networks
Download
Paper & Supplements
Hand Histories
Members (Front-back)
Michael Bowling, Dustin Morrill, Nolan Bard, Trevor Davis, Kevin Waugh, Michael Johanson, Viliam Lisý, Martin Schmid, Matej Moravčík, Neil Burch
low-variance Evaluation
The performance of DeepStack and its opponents was evaluated using AIVAT, a provably unbiased low-variance technique based on carefully constructed control variates. Thanks to this technique, which gives an unbiased performance estimate with 85% reduction in standard deviation, we can show statistical significance in matches with as few as 3,000 games.
Abstraction-based Approaches
Despite using ideas from abstraction, DeepStack is fundamentally different from abstraction-based approaches, which compute and store a strategy prior to play. While DeepStack restricts the number of actions in its lookahead trees, it has no need for explicit abstraction as each re-solve starts from the actual public state, meaning DeepStack always perfectly understands the current situation.
Professional Matches
We evaluated DeepStack by playing it against a pool of professional poker players recruited by the International Federation of Poker. 44,852 games were played by 33 players from 17 countries. Eleven players completed the requested 3,000 games with DeepStack beating all but one by a statistically-significant margin. Over all games played, DeepStack outperformed players by over four standard deviations from zero.
Heuristic Search
At a conceptual level, DeepStack’s continual re-solving, “intuitive” local search and sparse lookahead trees describe heuristic search, which is responsible for many AI successes in perfect information games. Until DeepStack, no theoretically sound application of heuristic search was known in imperfect information games.
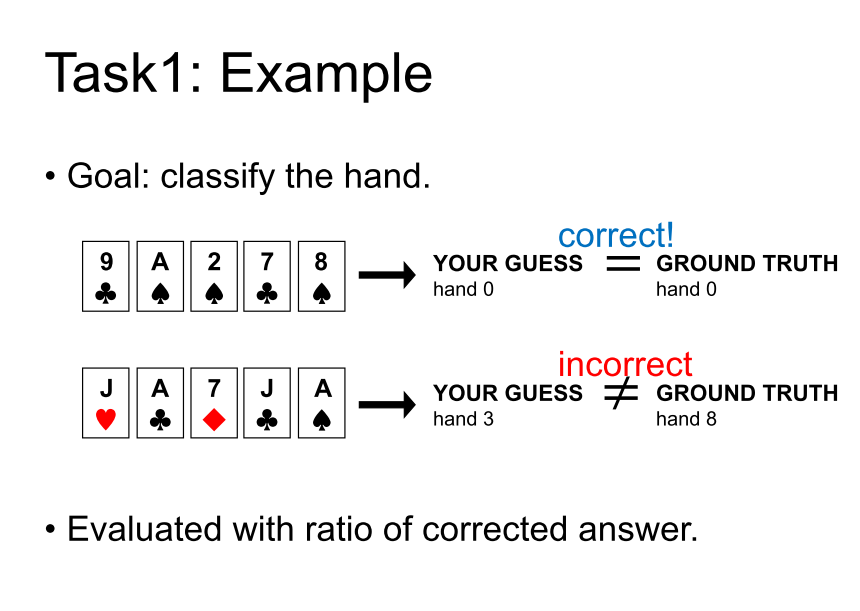
DeepStack, one of many recent computers to face off against human beings, defeated 11 professional poker players in heads-up no-limit hold’em, according to a study published in Science this month.
Of the 11 players, DeepStack defeated 10 of them in December 2016 by statistically significant margins after the study authors had the computer undergo deep learning training to teach the bot to develop poker intuition for any situation.
The computer looked up two copies of the same network in its neural network, namely for the first three shared cards and then again for the final two, trained on 10,000 randomly drawn poker games, reported Ars Technica.
The researchers recruited 33 players through the International Federation of Poker.
Only 11 players finished 3,000 matches over the course of a four-week period and DeepStack’s neural networks were what allowed it to essentially “learn” and model higher-level concepts while it ran on a gaming laptop (NVIDIA GTX 1080). DeepStack was developed by researchers at the University of Alberta and a number of Czech universities.
DeepStack works through situations as humans would, learning pieces of the game as it goes and create a strategy to defeat the humans.
“In some sense this is probably a lot closer to what humans do,” said Michael Bowling, professor of machine learning and the study author, to Scientific American. “Humans certainly don’t, before they sit down and play, precompute how they’re going to play in every situation. And at the same time, humans can’t reason through all the ways the poker game would play out all the way to the end.”
DeepStack isn’t the only artificial intelligence out there. Carnegie Mellon’s Libratus recently beat four professional players with a more elite status on a supercomputer. Its technology is similar to that of DeepStack in the later stages of computing but it does not use the same neural networks, according to Scientific American. DeepStack also won by larger margins.
Past attempts with Claudico didn’t pan out, but Google DeepMind’s Alpha Go beat pros at the game, go. Even more notably, 20 years ago, Deep Blue beat World Chess Champion Garry Kasparov at his own game.
This finding reveals a lot about artificial intelligence’s ability to master imperfect information games beyond abstraction (or computing how to play in every situation before the game begins).
Lead image courtesy of Thigala shri/Flickr
Poker Bot Neural Network
Tags
Poker PlayersAI